top of page
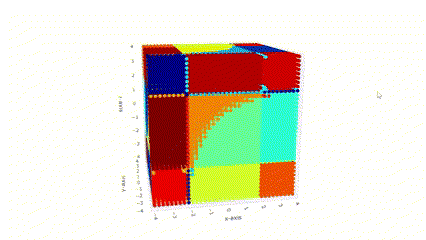
Research
I am interested in questions about the intersection of Algebraic Geometry, Statistics, and Machine Learning. The main goal is to understand the geometry of the model space in each particular setting from the noisy data.
-
For Machine Learning, we fix a neural network architecture and ambient space where our neural network lives. A neuromaniofold is a subspace of the ambient space of all possible neural networks of a fixed architecture. The goal is to understand the geometry of this neuromanifold.
-
For Algebraic Statistics, we consider Directed Graphical Models with or without cycles. These models can be encoded as an algebraic variety. The goal is to find defining equations for this variety.
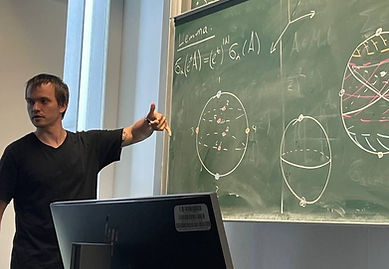
Preprints
-
Geometry of Rational Neural Networks
-
T. Boege, J. Selover and M. Zubkov: Sign patterns of principal minors of real symmetric matrices, arXiv:2407.17826.
Publications
-
Likelihood Geometry of Determinantal Point Processes (with Hannah Friedman and Bernd Sturmfels) submitted
-
Chromatic Graph Homology for Brace Algebras (with Vladimir Baranovsky) published in New York J. Math. 23 (2017) 1307–1319​
bottom of page